Abstract
Aims
The aim of this study was to evaluate the performance of metagenomic next-generation sequencing (mNGS) in detecting pathogens from synovial fluid of prosthetic joint infection (PJI) patients.
Methods
A group of 75 patients who underwent revision knee or hip arthroplasties were enrolled prospectively. Ten patients with primary arthroplasties were included as negative controls. Synovial fluid was collected for mNGS analysis. Optimal thresholds were determined to distinguish pathogens from background microbes. Synovial fluid, tissue, and sonicate fluid were obtained for culture.
Results
A total of 49 PJI and 21 noninfection patients were finally included. Of the 39 culture-positive PJI cases, mNGS results were positive in 37 patients (94.9%), and were consistent with culture results at the genus level in 32 patients (86.5%) and at the species level in 27 patients (73.0%). Metagenomic next-generation sequencing additionally identified 15 pathogens from five culture-positive and all ten culture-negative PJI cases, and even one pathogen from one noninfection patient, while yielding no positive findings in any primary arthroplasty. However, seven pathogens identified by culture were missed by mNGS. The sensitivity of mNGS for diagnosing PJI was 95.9%, which was significantly higher than that of comprehensive culture (79.6%; p = 0.014). The specificity is similar between mNGS and comprehensive culture (95.2% and 95.2%, respectively; p = 1.0).
Conclusion
Metagenomic next-generation sequencing can effectively identify pathogens from synovial fluid of PJI patients, and demonstrates high accuracy in diagnosing PJI.
Cite this article: Bone Joint Res 2020;9(7):440–449.
Article focus
-
Evaluate the sensitivity of metagenomic next-generation sequencing (mNGS) in detecting infecting microorganisms in synovial fluid.
-
Determine the accuracy of mNGS by assessing the concordance of its results compared to traditional culture techniques.
Key messages
-
Metagenomic next-generation sequencing exhibits both high sensitivity and specificity in diagnosing prosthetic joint infection (PJI).
-
Metagenomic next-generation sequencing may be an adjunct molecular diagnostic tool for identifying the pathogens of PJI, especially for culture-negative patients.
Strengths and limitations
-
Prospectively evaluating the diagnostic performance of mNGS in identifying pathogens from PJIs.
-
Relatively small volume of subjects.
-
Determination of the optimal thresholds for test interpretation can affect the sensitivity and specificity of mNGS.
Introduction
Identification of the infecting pathogen is critical to the successful management of prosthetic joint infections (PJIs).1 Currently, microbial culture is the principal diagnostic test for determining the infecting microorganism.2 However, despite enhancement strategies to improve the detection rate (i.e. sonication,3 prolonged incubation,4 and enhanced culture media),5 culture-negative infections remain a significant clinical challenge.6,7
Culture-independent techniques including polymerase chain reaction (PCR) have been used to improve the diagnostic accuracy of infecting organisms. However, multiplex PCR has failed to identify pathogens beyond a predesigned panel, and has demonstrated limited sensitivity ranging from 50.0% to 81.6%.8-10 Furthermore, while 16S ribosomal RNA gene PCR has the potential to detect the majority of bacteria, it is unable to identify fungal or polymicrobial infections and differentiate contaminants from the true infecting organism.11
Next-generation sequencing (NGS) is a novel and cost-effective technique that can identify all nucleic acids in a given specimen within a short time period. Amplicon-based NGS (aNGS) is able to sequence broad-range PCR amplicons in tandem,12 allowing efficient identification of both bacteria and fungi genomes. Tarabichi et al13 showed the utility of this technique by identifying potential pathogens from 81.9% of culture-negative PJI cases. Metagenomic next-generation sequencing (mNGS) has a further advantage over aNGS in that it is able to give a full-scale profile of microbes without targeted preamplification.14 Metagenomic next-generation sequencing has been shown to substantially increase the detection rate of microorganisms in infectious cerebrospinal fluid, bloodstreams, and respiratory systems.15-17
Studies using mNGS in PJI have been traditionally performed on explanted and sonicated prostheses. Thoendel et al18 demonstrated that this technique was able to identify known pathogens in 94.8% of culture-positive PJI cases, while identifying new potential pathogens in 43.9% of culture-negative infections. Additionally, Street et al19 reported that mNGS on sonicated fluid achieved 88.0% sensitivity at the genus level. However, sonicate fluid can only be obtained after joint resection. There are currently few studies evaluating the efficacy of mNGS on synovial fluid. Ivy et al20 applied mNGS approach to synovial fluid from failed knee arthroplasties, yielding additional pathogens not detected by culture, both from culture-positive and culture-negative cases. However, the diagnostic performance of PJI was not evaluated in this retrospective research.
Therefore, the purpose of this study was to evaluate the sensitivity of mNGS in detecting infecting microorganisms in synovial fluid, and determine the accuracy of this technique by assessing the concordance of its results compared to traditional culture techniques.
Methods
We prospectively enrolled 75 consecutive patients who underwent revision hip or knee procedures at our institution between March 2017 and July 2018. There were 41 men and 34 women with a mean age of 65.6 years (28 to 90). The indications for revision included PJI, loosening, instability, and periprosthetic fracture. A total of 16 patients underwent irrigation and debridement for persistent wound drainage following total hip arthroplasty (THA)/total knee arthroplasty (TKA) (less than four weeks), and late, acute haematogenous infection. PJI was defined according to the criteria of the Musculoskeletal Infection Society (MSIS).21 A cohort of patients undergoing primary THA/TKA (n = 10) with no history of inflammatory arthritis, joint infections, or prior surgical procedures were included as negative controls. Patients with incomplete clinical records which prevented differentiation of infection/noninfection, insufficient synovial fluid volume (< 1 ml), or sequencing failure were excluded. Sequencing failure is defined as the inability to adequately perform a complete sequencing analysis secondary to the insufficient DNA extraction or failed sequencing library conduction. The study was approved and conducted according to the guidelines set forth by our institutional review board (Protocol # 2017-094 and 2018-026). Because of inclusion of aseptic revisions and control primary arthroplasties, additional safeguards including the formation of a multidisciplinary team of microbiologists, infection disease specialists, and orthopaedic surgeons were put in place in the event of an unexpected positive mNGS result. All patients were followed for a minimum of 12 months.
Collection of synovial fluid and periprosthetic tissues
Preoperative aspiration was performed in all cases with abnormal preoperative ESR (Alifax, Genoa, Italy) and CRP (Beckman Coulter, Brea, California, USA). For patients with normal ESR and CRP, intraoperative arthrocentesis was performed prior to capsulotomy in order to avoid blood contamination. An aliquot of 0.5 ml of synovial fluid was assessed for white blood cell count and white blood cell differential (Sysmex, Hyogo, Japan). Another aliquot of 1 ml of synovial fluid was stored in DNase- and RNase-free sterile cryogenic vials (Axygen Scientific, Union City, California, USA) at -80°C for mNGS analysis. Residual fluid was used for culture. In all cases, a minimum of five periprosthetic tissue samples were collected after arthrotomy from sites with the most obvious inflammation for histopathological examination and culture. A positive culture was defined as the same organism isolated from a minimum of two cultures. All aerobic cultures were held for six days, and all anaerobic cultures were held for prolonged incubation for at least 14 days. The detailed microbiological culture procedure is outlined in Supplementary Material.
Implant sonication
We followed the procedure and workflow of implant sonication as described by Huang et al.11 This process included placing the removed implants in a sterile polypropylene sealable container, submerging the implants into 500 ml saline solution (Chimin Health Management, Taizhou, China), and spinning samples for 30 seconds followed by sonication for three minutes at 40 kHz (Woxing, Wuxi, China) in a sterile water bath. Each 50 ml aliquot of sonicate fluid was concentrated to a final volume of 1 ml by centrifugation (1,490 g for ten minutes). The resulting fluid was used for culture.
DNA extraction, library preparation, and sequencing
The extracted total genomical DNA was sonicated to generate fragments. DNA libraries were constructed through end-repairing, specific-adaptors ligating, purification, amplification by PCR, and generation of single-stranded DNA circles by circularization reactions. The quantified libraries were processed for 50 bp single-end sequencing on the BGISEQ-500 platform (BGI-Wuhan, Wuhan, China). Samples were processed in batches with a negative control (sterile saline).
Bioinformatics pipeline
The raw sequencing data were analyzed using a bioinformatics pipeline developed by BGI (Figure 1). The procedure briefly includes the following steps: 1) clean reads of high quality generated by filtering out short, low-quality, and low-complexity reads; 2) human host sequences eliminated by mapping to the human reference genome; and 3) alignment of the remaining sequencing data to the in-house-built microbial genome database, generating an original mapped list for advanced interpretation. The detailed workflow is listed in Supplementary Figure a.
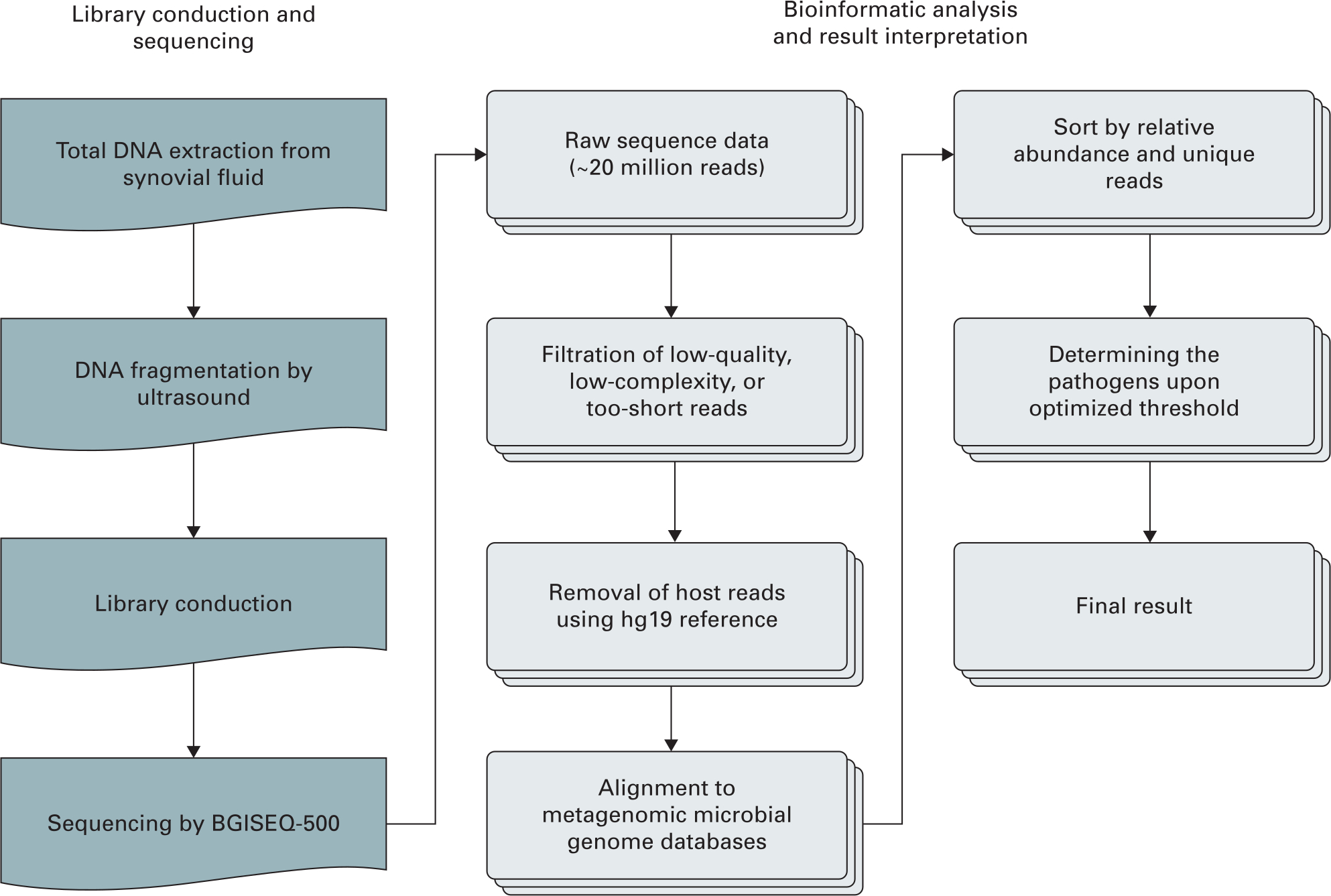
Fig. 1
General workflow of metagenomic next-generation sequencing. hg19, human reference genome.
Interpretation of mNGS result
The final result of mNGS was interpreted mainly using the number of unique reads, relative abundance, and genomical coverage rate mapping to pathogens. The detailed definition and calculation are listed in Supplementary Figure a. Optimal thresholds for bacterial and fungal identification were calculated individually. We determined the 15.0% relative abundance in genus level as the threshold for bacterial identification that hold the highest Youden index as 0.83, with the sensitivity (87.8%; 95% confidence interval (CI) 75.8% to 94.3%) and specificity (95.2%; 95% CI 77.3% to 99.2%) (Figures 2a and 2b). Additionally, the fungal isolates were relatively rare in this cohort. So, we used the 30.0% relative abundance in genus level as the threshold for fungi validated by Li et al22 using the same mNGS platform (Figure 2c).

Fig. 2
Optimal thresholds for infectious bacteria and fungi identification by metagenomic next-generation sequencing (mNGS). a) Youden index was calculated under different thresholds of relative abundance in genus level. The optimal threshold of bacterial relative abundance in genus level was determined as 15% with highest Youden index. b) Standardized number of reads stringently mapped to pathogen in genus level (SNRSMG) ≥ 3 were recognized as basic inclusive criteria. The graph shows the SNRSMG and relative abundance of bacteria genus (excluding Mycobacteria and Burkholderia), which were interpreted as potential pathogens by mNGS. Red dots indicate culture-positive bacteria identified by mNGS. Blue dots indicate bacteria genera that contained the highest relative abundance in samples of culture-negative prosthetic joint infection. Black dots indicate bacteria genera that contained the highest relative abundance in samples from the noninfection group. The vertical dotted line indicates the optimal threshold of bacterial relative abundance in genus level was determined as 15%. c) SNRSMG and relative abundance of fungal genera that were interpreted as potential pathogens by mNGS. Red dots indicate culture-positive fungi identified by mNGS. The vertical dotted line indicates the optimal threshold of fungal relative abundance in genus level was determined as 30%.
Statistical analysis
We performed an a priori power analysis to determine the minimum sample size necessary to achieve statistical significance. Using previously published data, we estimated the sensitivity of microbiological culture at 70.0% and mNGS at 90.0%.19 We determined that a sample size of 39 patients would achieve a power of 80.0% with an alpha error of 0.05. All clinical and laboratory data were analyzed using the non-parametric Mann-Whitney U test, the chi-squared test, and the independent-samples t-test. The sensitivities and specificities of microbiological tests were compared using the McNemar’s test for related proportions. In addition, a best- and worst-case scenario analysis including and excluding patients with failed sequencing (n = 2) was performed in order to minimize error. A p-value < 0.05 was considered significant. All analyses were performed using the SPSS v21.0 software (IBM, Armonk, New York, USA).
Results
Of the 75 patients enrolled, there were five exclusions: one patient with incomplete clinical data, two patients with insufficient synovial fluid, and two patients in which sequencing failed (one culture-negative PJI (CN-PJI) and one aseptic). Of the remaining 70 patients (36 THAs/34 TKAs), 49 were classified as infected according to the MSIS criteria and 21 were aseptic. The clinical and laboratory characteristics of enrolled patients are listed in Table I. The negative primary arthroplasty controls (five THAs/five TKAs) were classified as the primary arthroplasty control group (Figure 3a). At latest evaluation, there were no cases of delayed infection diagnosis in either the aseptic noninfected revision or primary arthroplasty control groups.
Table I.
Clinical and laboratory characteristics of study subjects.
Characteristic | Prosthetic joint infection (n = 49) | Noninfection (n = 21) | p-value |
---|---|---|---|
Mean age, yrs (SD) | 66.0 (13.8) | 64.9 (12.2) | 0.739* |
Sex, n (%) | 0.156† | ||
Male | 30 (61.2) | 9 (42.9) | |
Female | 19 (39.8) | 12 (57.1) | |
Site of arthroplasty, n (%) | 0.676† | ||
Knee | 26 (53.1) | 10 (47.6) | |
Hip | 23 (46.9) | 11 (52.4) | |
Mean body mass index, kg/m2 (SD) | 27.6 (4.8) | 28.3 (4.8) | 0.537* |
Surgical procedure, n (%) | 0.525† | ||
Debridement with implant retention | 12 (24.5) | 3 (14.3) | |
Revision with implant resection | 37(75.5) | 18 (85.7) | |
Antibiotics received two weeks prior to surgery, n (%) | 18 (36.7) | 4 (19.0) | 0.144† |
Clinical and laboratory findings | |||
Presence of sinus tract, n (%) | 12 (22.6) | 0 (0.0) | < 0.001† |
Positive synovial fluid,≥ two periprosthetic tissue cultures, n (%) | 36 (73.5) | 1 (4.8) | < 0.001† |
Mean synovial fluid white blood-cell count, cells/ml (SD) | 58,780.8 (101176.1) | 906.5 (548.3) | 0.011‡ |
Mean synovial fluid polymorphonuclear neutrophils, % (SD) | 79.7 (13.0) | 44.4 (17.0) | < 0.001‡ |
Mean serum CRP, mg/l (SD) | 47.4 (41.4) | 9.6 (7.1) | < 0.001‡ |
Mean serum ESR, mm/hr (SD) | 67.6 (34.2) | 13.5 (5.6) | < 0.001‡ |
Acute inflammation in periprosthetic tissue histopathology, n (%) | 24 (75.0) | 0 (0) | < 0.001† |
-
*
Independent-samples t-test.
-
†
Chi-squared test.
-
‡
Mann-Whitney U test.
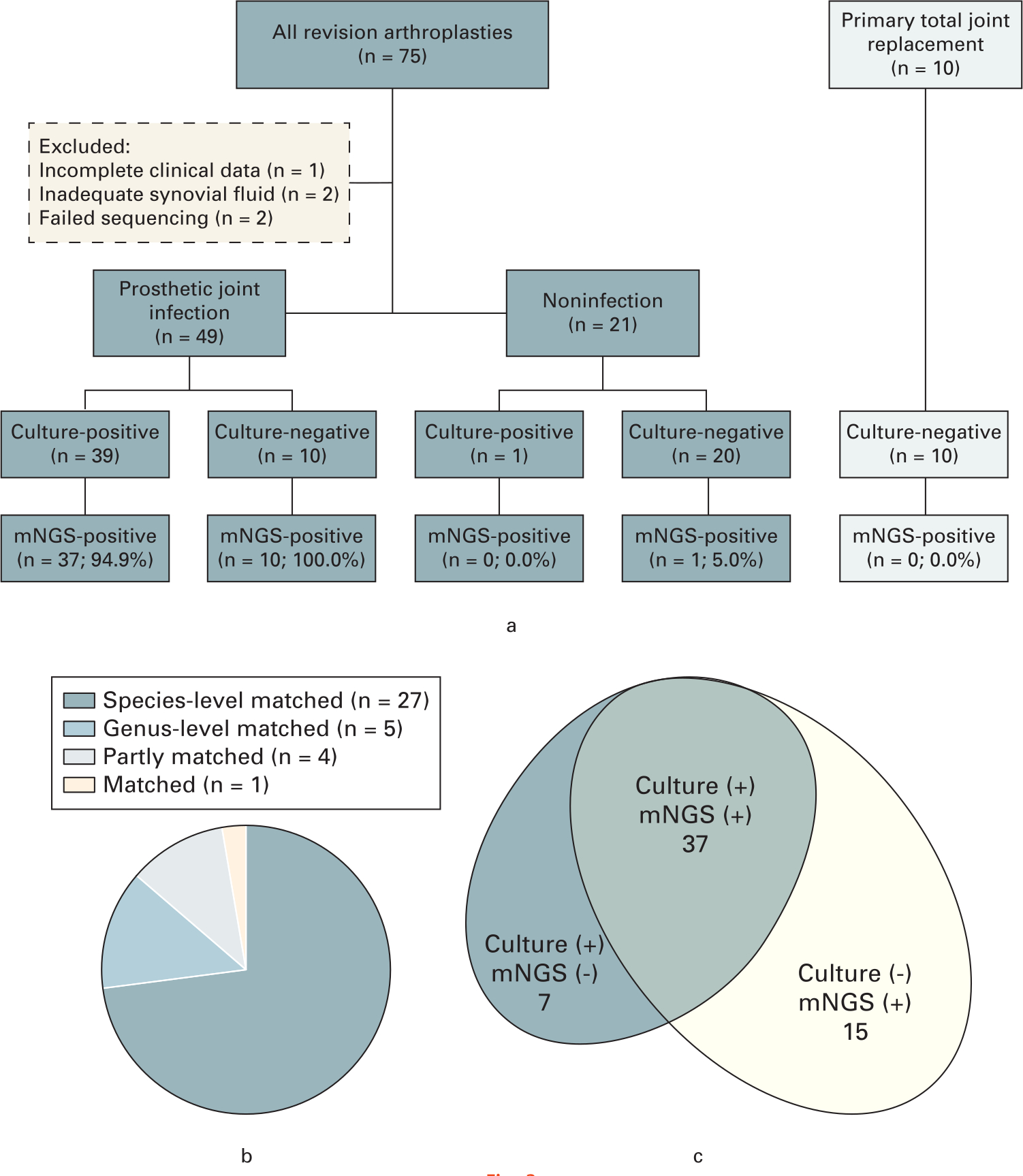
Fig. 3
a) Flowchart detailing enrolment and microbiological results of study samples. b) The concordance between metagenomic next-generation sequencing (mNGS) and culture of culture-positive prosthetic joint infection (PJI), where n = number of patients. c) The number of pathogens detected by mNGS in genus-level compared to culture in the PJI group.
Among the 49 infections, the causative pathogen was isolated in synovial fluid alone in 30 cases (61.2%), and the pathogen was isolated in comprehensive synovial, tissue, and sonicate fluid cultures in 39 patients (79.6%). These patients were allocated to the culture-positive PJI (CP-PJI) subgroup while the remaining ten PJI patients, who according to MSIS criteria had a negative comprehensive culture, were classified into the CN-PJI subgroup. The antibiotic regimen was determined by a multidisciplinary team of specialists based on the combination of culture and mNGS results. A single patient in the noninfected group had a single positive culture of coagulase-negative staphylococcus (CoNS) from the sonicate fluid. The patient was treated as aseptic failure without postoperative administration of antibiotic, and at last follow-up there were no clinical signs of infection. There were no positive cultures from the primary arthroplasty group. A single pathogen was isolated in 35 CP-PJI cases while four patients in this group had polymicrobial infections. The prevalence of infecting organisms in this cohort of patients is listed in Table II.
Table II.
Microbiology finding of synovial fluid culture, periprosthetic tissue culture, comprehensive culture, and synovial fluid metagenomic next-generation sequencing.
Microorganism | No. of isolates from synovial fluid culture | No. of isolates from periprosthetic tissue | No. of isolates from comprehensive culture | No. of identifications from synovial fluid by mNGS |
---|---|---|---|---|
Coagulase-negative staphylococci | 10 | 10 | 13 | 13 |
Staphylococcus aureus | 7 | 8 | 8 | 8 |
Anaerobe* | 2 | 2 | 2 | 5 |
Streptococci | 5 | 5 | 5 | 6 |
Gram-negative bacilli | 3 | 3 | 4 | 5 |
Mycoplasma † | 0 | 0 | 0 | 4 |
Enterococcus faecalis | 3 | 3 | 5 | 3 |
Candida ‡ | 3 | 5 | 5 | 4 |
Mycobacterium | 0 | 1 | 1 | 2 |
Other organisms§ | 1 | 2 | 2 | 3 |
Total | 34 | 39 | 45 | 53 |
-
*
Including Parvimonas micra, Bacteroides fragilis, and Cutibacterium acnes.
-
†
Including Mycoplasma hominis.
-
‡
Including Candida albicans, Candida parapsilosis, and Candida tropicalis.
-
§
Including Neisseria spp., Helcococcus kunzii, and Corynebacterium tuberculostearicum.
-
mNGS, metagenomic next-generation sequencing.
The cost of mNGS per case was $300 USD and took 48 hours to generate an interpreted result. A median 24,221,983 raw reads (interquartile range (IQR) 18,136,658 to 27,783,216) were generated from each sequencing. The numbers of total reads from the PJI, noninfection, and primary arthroplasty groups were similar (p = 0.058, non-parametric Kruskal-Wallis test; Figure 4). The mNGS signals in the primary arthroplasty group were mostly very weak including low number of reads and low coverage rate of the genome. Most of the mapped microbes were similar among the different control samples considered to be background signals from reagents or the laboratory environment. The raw mapping result of mNGS always provided a wide range of microbial profiles that included pathogens as well as background microorganisms.
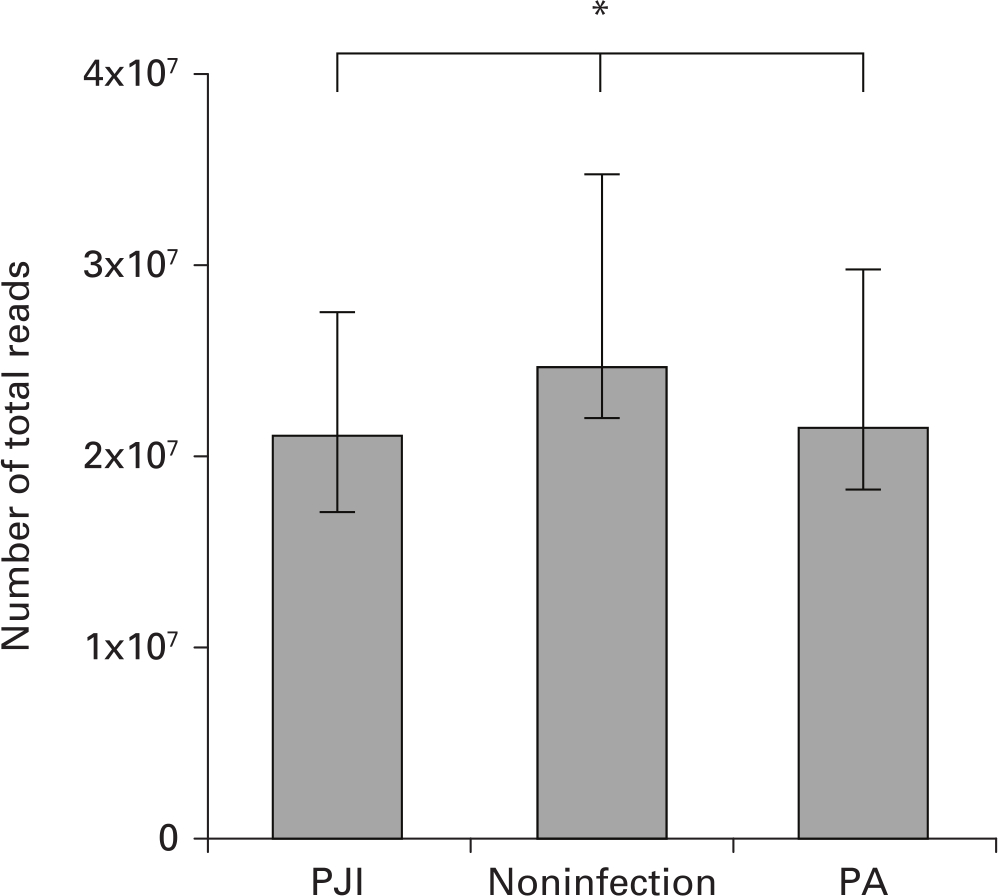
Fig. 4
Comparison of median number of mapped reads among prosthetic joint infection (PJI), noninfection, and primary arthroplasty (PA) groups. Bars indicate interquartile ranges of reads. *p = 0.058
Of 39 CP-PJI cases, mNGS applied to the synovial fluid was able to detect positive pathogens concordant with comprehensive culture results in 37 patients (94.9%). Furthermore, mNGS results were completely consistent at the genus level in 32 PJI patients (86.5%), at the species level in 27 PJIs (73.0%), partly inconsistent in four PJIs (10.8%), and totally inconsistent in one PJI (2.9%) (Figure 3b). Metagenomic next-generation sequencing detected additional potential pathogens in five CP-PJI patients, including Mycobacterium tuberculosis (n = 1), Cutibacterium acnes (n = 2), Shigella dysenteriae (n = 1), and Mycoplasma hominis (n = 1). In six CP-PJI cases, seven isolates were missed by mNGS including staphylococci (n = 3), Acinetobacter baumannii (n = 1), Enterococcus faecalis (n = 2), and Candida tropicalis (n = 1). Finally, in all ten CN-PJI cases, mNGS was able to detect potential pathogens including staphylococci (n = 3), Mycoplasma hominis (n = 3), Parvimonas micra (n = 1), streptococci (n = 1), Varibaculum (n = 1), and Corynebacterium (n = 1) (Figure 3c). With a minimum follow-up of 12 months, there were no recurrent infections in the CP-PJI and CN-PJI groups.
Using the MSIS definition for infection as the benchmark, the sensitivity of mNGS in synovial fluid was 95.9%, significantly higher than the sensitivities observed for synovial fluid culture (61.2%; p < 0.001, chi-squared test) or comprehensive cultures (79.6%; p = 0.014, chi-squared test). While there were no statistically significant differences in specificity among synovial fluid culture, comprehensive culture, and synovial fluid mNGS (100.0%, 95.2%, and 95.2%, respectively; p = 0.100, chi-squared test), the sensitivity reached 98.0% when mNGS was combined with microbiological culture of synovial fluid specimen and 100.0% when combined with comprehensive cultures. Meanwhile, the specificity achieved by both combinations remained high (95.2% and 90.5%, respectively) (Table III). Finally, a best- and worst-case scenario analysis including the two patients censored for sequencing failure as either CN-PJI (n = 1) or aseptic failure (n = 1) still showed higher sensitivity of synovial mNGS compared to comprehensive culture (p = 0.045, chi-squared test) (Tables IV and V).
Table III.
Comparison of diagnostic value among different microbiological tests, in which two sequencing failed patients had been excluded.
Positive microbiological test | Prosthetic joint infection (n = 49) | Noninfection (n = 21) | Sensitivity, % (95% CI) | Specificity, % (95% CI) | Positive predictive value, % (95% CI) | Negative predictive value, % (95% CI) |
---|---|---|---|---|---|---|
Synovial fluid culture | 30 | 0 | 61.2 (47.2 to 73.6) | 100.0 (84.5 to 100.0) | 100.0 (88.6 to 100.0) | 52.5 (37.5 to 67.1) |
Comprehensive culture | 39 | 1 | 79.6 (66.4 to 88.5) | 95.2 (77.3 to 99.2) | 97.5 (87.1 to 99.6) | 66.7 (48.8 to 80.8) |
Synovial fluid mNGS | 47 | 1 | 95.9 (86.3 to 98.9) | 95.2 (77.3 to 99.2) | 97.9 (89.1 to 99.6) | 90.9 (72.2 to 97.5) |
Synovial fluid culture or mNGS | 48 | 1 | 98.0 (89.3 to 99.6) | 95.2 (77.3 to 99.2) | 98.0 (89.3 to 99.6) | 95.2 (77.3 to 99.2) |
Comprehensive culture or synovial fluid mNGS | 49 | 2 | 100.0 (92.7 to 100.0) | 90.5 (71.1 to 97.3) | 96.1 (86.8 to 98.9) | 100.0 (83.2 to 100.0) |
-
CI, confidence interval; mNGS, metagenomic next-generation sequencing
Table IV.
Comparison of diagnostic value among different microbiological tests. This includes a best-case scenario analysis including the two patients censored for sequencing failure as either culture-negative prosthetic joint infection (n = 1) or noninfection (n = 1).
Positive microbiological test | Prosthetic joint infection (n = 50) | Noninfection (n = 22) | Sensitivity, % (95% CI) | Specificity, % (95% CI) | Positive predictive value, % (95% CI) | Negative predictive value, % (95% CI) |
---|---|---|---|---|---|---|
Synovial fluid culture | 30 | 0 | 60.0 (46.2 to 72.4) | 100.0 (85.1 to 100.0) | 100.0 (88.6 to 100.0) | 52.4 (37.7 to 66.6) |
Comprehensive culture | 39 | 1 | 78.0 (64.8 to 87.2) | 95.5 (78.2 to 99.2) | 97.5 (87.1 to 99.6) | 65.6 (48.3 to 79.6) |
Synovial fluid mNGS | 47 | 1 | 94.0 (83.8 to 97.9) | 95.5 (78.2 to 99.2) | 97.9 (89.1 to 99.6) | 87.5 (69 to 95.7) |
Synovial fluid culture or mNGS | 48 | 1 | 96.0 (86.5 to 98.9) | 95.5 (78.2 to 99.2) | 98.0 (89.3 to 99.6) | 91.3 (73.2 to 97.6) |
Comprehensive culture or synovial fluid mNGS | 49 | 2 | 98.0 (89.5 to 99.6) | 95.5 (78.2 to 99.2) | 98 (89.5 to 99.6) | 95.5 (78.2 to 99.2) |
-
CI, confidence interval; mNGS, metagenomic next-generation sequencing
Table V.
Comparison of diagnostic value among different microbiological tests. This includes a supposed worst-case scenario analysis including the two patients censored for sequencing failure as either culture-negative prosthetic joint infection (n = 1) or noninfection (n = 1).
Positive microbiological test | Prosthetic joint infection (n = 51) | Noninfection (n = 21) | Sensitivity, % (95% CI) | Specificity, % (95% CI) | Positive predictive value, % (95% CI) | Negative predictive value, % (95% CI) |
---|---|---|---|---|---|---|
Synovial fluid culture | 32 | 0 | 62.7 (49 to 74.7) | 100.0 (84.5 to 100.0) | 100.0 (89.3 to 100.0) | 52.5 (37.5 to 67.1) |
Comprehensive culture | 41 | 1 | 80.4 (67.5 to 89) | 95.2 (77.3 to 99.2) | 97.6 (87.7 to 99.6) | 66.7 (48.8 to 80.8) |
Synovial fluid mNGS | 47 | 1 | 92.2 (81.5 to 96.9) | 95.2 (77.3 to 99.2) | 97.9 (89.1 to 99.6) | 83.3 (64.1 to 93.3) |
Synovial fluid culture or mNGS | 48 | 1 | 94.1 (84.1 to 98.0) | 95.2 (77.3 to 99.2) | 98.0 (89.3 to 99.6) | 87.0 (67.9 to 95.5) |
Comprehensive culture or synovial fluid mNGS | 51 | 2 | 100.0 (93.0 to 100.0) | 90.5 (71.1 to 97.3) | 96.2 (87.2 to 99.0) | 100.0 (83.2 to 100.0) |
-
CI, confidence interval; mNGS, metagenomic next-generation sequencing
Discussion
Accurate identification of infecting organisms in PJI of the hip and knee joints can improve the rate of infection control.1 Conventional culture methods, even with sonication, can still result in a substantial number of culture-negative infections that are barriers to proper antibiotic selection.3 As a novel molecular technique, mNGS has the ability to obtain accurate and complete genome-level profiles of pathogens in a single, rapid, and cost-effective assay. This diagnostic technique has been validated in sonicates from explanted prostheses but has not been prospectively studied in the setting of synovial fluid aspirates prior to revision joint arthroplasty. We previously reported our mNGS process and workflow and demonstrated that it can identify infecting pathogens in synovial fluid in a case of culture-negative infection.23 Our results from this show that mNGS can detect pathogenic bacteria from synovial fluid as effectively as sonicate fluid, especially in culture-negative infection cases.
Our study has several limitations. Firstly, although the sample size was sufficiently powered, the number of hip and knee infections included in our pilot study was relatively low and could have affected the reliability of the results. However, the inclusion of various levels of controls (i.e. aseptic revisions and even native joints) and correlation with conventional culture results can reduce the number of false positives and negatives. Furthermore, the addition of best- and worst-case scenario analyses minimizes type II error. Secondly, our determination of the optimal thresholds for test interpretation can affect the sensitivity and specificity of mNGS. We acknowledge that there is no validated protocol for the determination of such a threshold, and as a result the decision to set this level of detection and assign certain pathogens as contaminants is arbitrary but based on our institutional laboratory experience. Additional studies from other laboratory centres are necessary to determine the optimal thresholds and determination of contaminants. Finally, mNGS failed to detect seven pathogens in cases of culture-confirmed PJI. Ivy et al20 similarly found mNGS was unable to identify pathogens in 14 culture-positive PJI cases. The cause of this discordant result is unexpected, concerning, and likely multifactorial but highlights the need for additional testing refinements. The majority of these pathogens were gram-positive cocci and fungi. While we used bead-beating to enhance DNA extraction, our yield of DNA was lower in gram-positive cocci and fungi compared to bacillus. Similar to other molecular diagnostic methods, mNGS requires strict storage and transport conditions to decrease nucleic acid degradation. Insufficient DNA extraction can lead to a low number of reads or insufficient genome coverage in the setting of greater background reads. Additional methods such as enzymatic disruption of cell wall, increasing sequencing depth, and refining detection thresholds can potentially mitigate this problem.
In this series, most of the pathogens in culture-positive infections were also identified by mNGS, reaching a genus-level consistency at 86.5% and species-level consistency at 73.0%. In some ways, this result is somewhat surprising since one would think that the concordance would be higher if the mNGS is a sensitive and specific test for the diagnosis of PJI. Several reasons can account for this discrepancy. Firstly, pitfalls in processing, establishment of background thresholds, and nucleic acid isolation can lead to failure to identify an organism and/or insufficient genome coverage to allow for accurate mapping to a species level. Secondly, the manifestation of PJI can be highly variable and the presence of other infecting organisms and the host microbiome can influence the relative abundances of various pathogens. Weaver et al24 also showed that discordance between genomical analysis and culture results was possible and reported positive cultures of organisms falling below their whole genome shotgun sequencing thresholds. Furthermore, mNGS also identified five additional pathogens from CP-PJI cases, as well as ten pathogens from CN-PJI cases. The technique was most useful in identifying organisms that usually require special culture conditions such as Mycoplasma,25Mycobacterium,26 and C. acnes.27 The test was also helpful in the diagnosis and identification of pathogens (streptococci and staphylococci) in cases that had received antibiotics within two weeks prior to resection arthroplasty. These results are consistent with prior reports using molecular techniques of pathogen detection. Miao et al28 analyzed 511 various types of samples from suspected infected patients in the clinical setting, and reported the sensitivity of mNGS as superior to that of culture (52.5% vs 34.2%; p < 0.01) in cases with antibiotic exposure. Furthermore, Thoendel et al18 reported detection of additional pathogens in 9.6% (11/115) of culture-positive PJI specimens, which were considered potential polymicrobial infections. In our cases, modifications of antimicrobial therapy according to the additional microbiological findings of mNGS results led to good infection control and no treatment failures at last follow-up. Consequently, mNGS can be a helpful adjunct in the diagnosis of PJI, in particular cases with difficulty in pathogen isolation in those with recent history of antibiotic use.
Overall, the sensitivity of mNGS in this pilot study to evaluate the diagnostic potential of this method in synovial fluid was 91.8%, significantly higher than a comprehensive culture method. Even when including the censored patients for sequencing failure, the sensitivity of mNGS was still higher compared to culture results. This outcome is comparable to the results reported from previous investigations of pathogens detected from sonicate fluid by mNGS.18,19 However, the mNGS technique applied in this study was more efficient and concise, and was devoid of microbial nucleic acid enrichment and whole genomical amplification,29,30 which saved time, labour, and costs. The current strategy can also reduce the risk of contaminant microbial DNA being introduced by the additional steps and reagents.30 When combing mNGS and culture tests on synovial fluid, there was only a single PJI without an identified pathogen. The clinical implication is the ability to not only diagnose infection accurately, but also to determine a causative microorganism(s) prior to surgery which can help guide treatment (i.e. irrigation and debridement or single-stage exchanges) and antimicrobial strategies both intraoperatively (i.e. spacer fabrication) and postoperatively. Consequently, more accurate treatment regimens could be developed to improve the prognosis and reduce the number of complications.
The principal challenge of molecular pathogen detection in PJI rests on our ability to identify true infecting microorganisms while disregarding environmental contaminants and normal microbiota. These results show that while mNGS is a powerful molecular detection tool, it has limitations and should not be used as a standalone test in the diagnosis of PJI. Metagenomic next-generation sequencing leverages the advantage of quantitative analysis to help distinguish real pathogens from background contaminants.31 However, the preamplification targeting of 16S ribosomal RNA is more complex, prone to contamination by environmental species, and can create a bias towards the actual composition of microorganisms.32 Since our technique did not include enrichment of nucleic acids, the number of reads mapping to pathogens may not reach a level to sufficiently contrast background reads. We therefore used relative rather than absolute mapping reads abundance values and followed the approach described by Street et al19 to determine optimal thresholds for pathogen detection. The optimal thresholds can be affected by laboratory workflow and environments, sequencing platforms, and specimen types. Each centre needs to adjust, calibrate, and validate their thresholds and environmental contaminant databases in order to ensure the validity of their results.31 However, long-term follow-up will still need to be determined if the pathogens that have been screened out are the real contaminants.
In conclusion, mNGS can effectively identify pathogens of synovial fluid in PJI patients, especially in those cases with negative cultures caused by either fastidious pathogens or recent antibiotic administration. The technique exhibits both high sensitivity and specificity but it is not perfect and should not be used as a standalone test. Combing mNGS with conventional microbiological cultures maximizes pathogen detection in the vast majority of PJI cases. Further study and refinement of the techniques and thresholds for distinguishing true infection pathogens from contaminants is needed to improve sensitivity and specificity.
References
1. Tzeng A , Tzeng TH , Vasdev S , et al. Treating periprosthetic joint infections as biofilms: key diagnosis and management strategies . Diagn Microbiol Infect Dis . 2015 ; 81 ( 3 ): 192 – 200 . Crossref PubMed Google Scholar
2. Della Valle C , Parvizi J , Bauer TW , et al. American Academy of Orthopaedic Surgeons. American Academy of Orthopaedic Surgeons clinical practice guideline on: the diagnosis of periprosthetic joint infections of the hip and knee . J Bone Joint Surg Am . 2011 ; 93-A ( 14 ): 1355 – 1357 . Google Scholar
3. Trampuz A , Piper KE , Jacobson MJ , et al. Sonication of removed hip and knee prostheses for diagnosis of infection . N Engl J Med . 2007 ; 357 ( 7 ): 654 – 663 . Crossref PubMed Google Scholar
4. Schäfer P , Fink B , Sandow D , et al. Prolonged bacterial culture to identify late periprosthetic joint infection: a promising strategy . Clin Infect Dis . 2008 ; 47 ( 11 ): 1403 – 1409 . Crossref PubMed Google Scholar
5. Shen H , Tang J , Wang Q , Jiang Y , Zhang X . Sonication of explanted prosthesis combined with incubation in BD bactec bottles for pathogen-based diagnosis of prosthetic joint infection . J Clin Microbiol . 2015 ; 53 ( 3 ): 777 – 781 . Crossref PubMed Google Scholar
6. Parvizi J , Erkocak OF , Della Valle CJ . Culture-negative periprosthetic joint infection . J Bone Joint Surg Am . 2014 ; 96-A ( 5 ): 430 – 436 . Crossref PubMed Google Scholar
7. Ahmed SS , Haddad FS . Prosthetic joint infection . Bone Joint Res . 2019 ; 8 ( 11 ): 570 – 572 . Crossref PubMed Google Scholar
8. Ryu SY , Greenwood-Quaintance KE , Hanssen AD , Mandrekar JN , Patel R . Low sensitivity of periprosthetic tissue PCR for prosthetic knee infection diagnosis . Diagn Microbiol Infect Dis . 2014 ; 79 ( 4 ): 448 – 453 . Crossref PubMed Google Scholar
9. Hischebeth GTR , Gravius S , Buhr JK , et al. Novel Diagnostics in Revision Arthroplasty: Implant Sonication and Multiplex Polymerase Chain Reaction . J Vis Exp . 2017 ; 130 : 1 – 8 . Crossref PubMed Google Scholar
10. Villa F , Toscano M , De Vecchi E , Bortolin M , Drago L . Reliability of a multiplex PCR system for diagnosis of early and late prosthetic joint infections before and after broth enrichment . Int J Med Microbiol . 2017 ; 307 ( 6 ): 363 – 370 . Crossref PubMed Google Scholar
11. Huang Z , Wu Q , Fang X , et al. Comparison of culture and broad-range polymerase chain reaction methods for diagnosing periprosthetic joint infection: analysis of joint fluid, periprosthetic tissue, and sonicated fluid . Int Orthop . 2018 ; 42 ( 9 ): 2035 – 2040 . Crossref PubMed Google Scholar
12. Chen MF , Chang CH , Chiang-Ni C , et al. Rapid analysis of bacterial composition in prosthetic joint infection by 16S rRNA metagenomic sequencing . Bone Joint Res . 2019 ; 8 ( 8 ): 367 – 377 . Crossref PubMed Google Scholar
13. Tarabichi M , Shohat N , Goswami K , et al. Diagnosis of Periprosthetic Joint Infection: The Potential of Next-Generation Sequencing . J Bone Joint Surg Am . 2018 ; 100-A ( 2 ): 147 – 154 . Crossref PubMed Google Scholar
14. Laudadio I , Fulci V , Palone F , et al. Quantitative Assessment of Shotgun Metagenomics and 16S rDNA Amplicon Sequencing in the Study of Human Gut Microbiome . OMICS . 2018 ; 22 ( 4 ): 248 – 254 . Crossref PubMed Google Scholar
15. Guan H , Shen A , Lv X , et al. Detection of virus in CSF from the cases with meningoencephalitis by next-generation sequencing . J Neurovirol . 2016 ; 22 ( 2 ): 240 – 245 . Crossref PubMed Google Scholar
16. Long Y , Zhang Y , Gong Y , et al. Diagnosis of Sepsis with Cell-free DNA by Next-Generation Sequencing Technology in ICU Patients . Arch Med Res . 2016 ; 47 ( 5 ): 365 – 371 . Crossref PubMed Google Scholar
17. Zinter MS , Dvorak CC , Mayday MY , et al. Pulmonary Metagenomic Sequencing Suggests Missed Infections in Immunocompromised Children . Clin Infect Dis . 2019 ; 68 ( 11 ): 1847 – 1855 . Crossref PubMed Google Scholar
18. Thoendel MJ , Jeraldo PR , Greenwood-Quaintance KE , et al. Identification of Prosthetic Joint Infection Pathogens Using a Shotgun Metagenomics Approach . Clin Infect Dis . 2018 ; 67 ( 9 ): 1333 – 1338 . Crossref PubMed Google Scholar
19. Street TL , Sanderson ND , Atkins BL , et al. Molecular Diagnosis of Orthopedic-Device-Related Infection Directly from Sonication Fluid by Metagenomic Sequencing . J Clin Microbiol . 2017 ; 55 ( 8 ): 2334 – 2347 . Crossref PubMed Google Scholar
20. Ivy MI , Thoendel MJ , Jeraldo PR , et al. Direct Detection and Identification of Prosthetic Joint Infection Pathogens in Synovial Fluid by Metagenomic Shotgun Sequencing . J Clin Microbiol . 2018 ; 56 ( 9 ): e00402 – e00418 . Crossref PubMed Google Scholar
21. Parvizi J , Zmistowski B , Berbari EF , et al. New definition for periprosthetic joint infection: from the Workgroup of the Musculoskeletal Infection Society . Clin Orthop Relat Res . 2011 ; 469 ( 11 ): 2992 – 2994 . Crossref PubMed Google Scholar
22. Li H , Gao H , Meng H , et al. Detection of Pulmonary Infectious Pathogens From Lung Biopsy Tissues by Metagenomic Next-Generation Sequencing . Front Cell Infect Microbiol . 2018 ; 8 : 205 . Crossref PubMed Google Scholar
23. Huang Z , Zhang C , Li W , et al. Metagenomic next-generation sequencing contribution in identifying prosthetic joint infection due to Parvimonas micra: a case report . J Bone Jt Infect . 2019 ; 4 ( 1 ): 50 – 55 . Crossref PubMed Google Scholar
24. Weaver AA , Hasan NA , Klaassen M , et al. Prosthetic joint infections present diverse and unique microbial communities using combined whole-genome shotgun sequencing and culturing methods . J Med Microbiol . 2019 ; 68 ( 10 ): 1507 – 1516 . Crossref PubMed Google Scholar
25. Tyner HL , Virk A , Nassr A , Razonable R . Mycoplasma hominis vertebral spine infection: case report and a review of infections of bone and joints . J Infect Chemother . 2016 ; 22 ( 11 ): 755 – 758 . Crossref PubMed Google Scholar
26. Huang Z , Zhang C , Fang X , et al. Identification of musculoskeletal infection with non-tuberculous Mycobacterium using metagenomic sequencing . J Infect . 2019 ; 78 ( 2 ): 158 – 169 . Crossref PubMed Google Scholar
27. Butler-Wu SM , Burns EM , Pottinger PS , et al. Optimization of periprosthetic culture for diagnosis of Propionibacterium acnes prosthetic joint infection . J Clin Microbiol . 2011 ; 49 ( 7 ): 2490 – 2495 . Crossref PubMed Google Scholar
28. Miao Q , Ma Y , Wang Q , et al. Microbiological Diagnostic Performance of Metagenomic Next-generation Sequencing When Applied to Clinical Practice . Clin Infect Dis . 2018 ; 67 ( suppl_2 ): S231 – S240 . Crossref PubMed Google Scholar
29. Thoendel M , Jeraldo PR , Greenwood-Quaintance KE , et al. Comparison of microbial DNA enrichment tools for metagenomic whole genome sequencing . J Microbiol Methods . 2016 ; 127 : 141 – 145 . Crossref PubMed Google Scholar
30. Thoendel M , Jeraldo P , Greenwood-Quaintance KE , et al. Impact of Contaminating DNA in Whole-Genome Amplification Kits Used for Metagenomic Shotgun Sequencing for Infection Diagnosis . J Clin Microbiol . 2017 ; 55 ( 6 ): 1789 – 1801 . Crossref PubMed Google Scholar
31. Schlaberg R , Chiu CY , Miller S , Procop GW , Weinstock G . Professional Practice Committee and Committee on Laboratory Practices of the American Society for Microbiology; Microbiology Resource Committee of the College of American Pathologists. Validation of Metagenomic Next-Generation Sequencing Tests for Universal Pathogen Detection . Arch Pathol Lab Med . 2017 ; 141 ( 6 ): 776 – 786 . Google Scholar
32. Boers SA , Jansen R , Hays JP . Understanding and overcoming the pitfalls and biases of next-generation sequencing (NGS) methods for use in the routine clinical microbiological diagnostic laboratory . Eur J Clin Microbiol Infect Dis . 2019 ; 38 ( 6 ): 1059 – 1070 . Crossref PubMed Google Scholar
Author contributions
Z. Huang: Designed the study, Collected and analyzed the data, Wrote the manuscript.
W. Li: Designed the study, Collected and analyzed the data, Wrote the manuscript.
G-C. Lee: Wrote and edited the manuscript.
X. Fang: Analyzed the data, Edited the manuscript.
L. Xing: Analyzed the data, Edited the manuscript.
B. Yang: Performed the microbiological culture experiments, Edited the manuscript.
J. Lin: Designed and supervised the study, Edited the manuscript.
W. Zhang: Designed and supervised the study, Edited the manuscript.
Funding statement
This work was supported by Fujian Education and Scientific Research Projects for Young Teachers (grant number JAT170241), National Science Foundation for Young Scientists of China (grant number 81702168), and Natural Science Foundation of Fujian Province (grant number 2018I0006, 2018Y4003, 2019I0011). Funds were used for laboratory testing fee and research coordinator salary.
No benefits in any form have been received or will be received from a commercial party related directly or indirectly to the subject of this article.
ICMJE COI statement
None declared.
Acknowledgements
Zida Huang and Wenbo Li are co-first authors and contributed equally to this work. We thank Jianying Yuan and Dong-ao Bi from BGI-Shenzhen for their contributions in performing metagenomic sequencing and bioinformatic analysis, Chongjing Zhang, Qijin Wang, and Mengqing Li for their contributions in sample collection, and Deqing Hu for his contributions in patient recruitment.
Ethical review statement
The study was approved and conducted according to the guidelines set forth by our institutional review board (Protocol # 2017 to 094 and 2018 to 026). Written informed consents were obtained from the patients included.
Supplementary material
Supplementary text, table, and figure describing the microbiological culture methods, process of next-generation sequencing, bioinformatic analysis, and interpretation of sequencing results applied in this study.
© 2020 Author(s) et al. This is an open-access article distributed under the terms of the Creative Commons Attribution Non-Commercial No Derivatives (CC BY-NC-ND 4.0) licence, which permits the copying and redistribution of the work only, and provided the original author and source are credited. See https://creativecommons.org/licenses/by-nc-nd/4.0/.